Will AI suffer from the things our real brains suffer from? Will it be as faulty and as vulnerable as real I?
I certainly hope not. But it stands to reason, if the human brain is creating a faster, stronger version of itself, how will our flawed brains ensure its flaws are left out of the new design?
I’m not sure there are elegant analogies in machine learning for ADHD, depression, anxiety, schizophrenia or epilepsy. But there is a characteristic of the human brain for which a direct analogy in AI exists, and that is bias. It is not quite a disorder per se, as bias exists in all of us. It is ugly and unavoidable in every day life. In an AI context, it can be as detrimental as it can be ubiquitous.
In the first season of True Detective, Nic Pizzolato’s critically-acclaimed series about the police investigations of horrific crimes, Detective Rust Cohle, often bleakly describing the meaninglessness of life to his partner Marty Hart, says “However illusory our identities are, we craft those identities by making value judgments. Everybody judges, all the time. Now, you got a problem with that, you’re living wrong.”
Our biases and value judgements are as old as humanity itself.
So how do we ensure we do not impregnate a device designed to think and learn with all the mistakes we ourselves make when we think and learn?
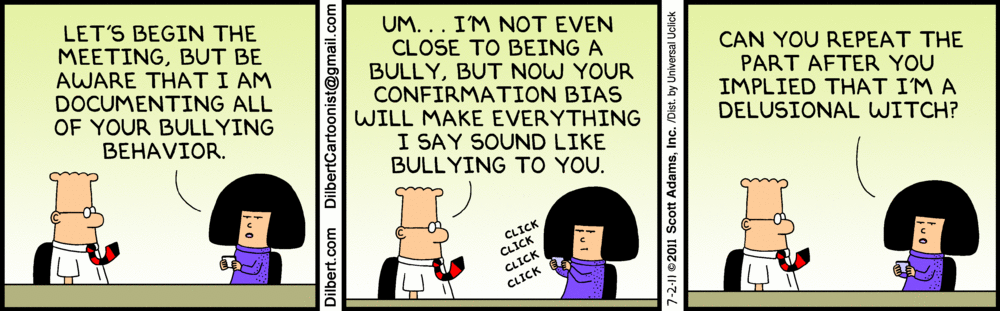
Before going any further, let’s get something straight: AI isn’t new. It’s been around since the 1950s. It also isn’t a thing. Rather, it’s a whole topic full of things. A quick search on Wikipedia yields the following definition, quoting Poole et al: “AI is the study of Intelligent agents, <defined as> any device that perceives its environment and takes actions that maximize its chance of successfully achieving its goals.” [1] In most applications, AI involves the use of algorithms that rely on a machine learning model to study data and find patterns or make predictions about future data. Most are also designed to continue learning as new data is assessed, which is what makes AI so compelling and so contentious at the same time.
It has become uber relevant mainly due to the newly available abundance of data. It is much more efficient to write an algorithm to sift through and organize a ton of data than it is for a human to do so. Still, human choices during the design of the model dictate the way the AI characterizes that data and guide the decision making and subsequent learning.
So, what could go wrong? Besides man-made robots turning on their makers and ironically destroying mankind, several little things that may seem inconsequential, or even go unnoticed, can lead to issues ranging from inconvenient to discriminatory to downright disastrous. On the discriminatory end of the spectrum, Laura Douglas, CEO of MyLevels, does a lovely job with the topic of gender bias in her blog post entitled “AI is not just learning our biases; it is amplifying them”. [2]
In the two contexts I can imagine the impact of bias being the most disastrous – AI-based medical diagnostic systems and AI-based driverless cars – the worst-case scenarios are easy to imagine: autonomous vehicles crashing into each other and running over people like the trucks in Stephen King’s Maximum Overdrive, and a medical system missing a life-threatening diagnosis or diagnosing disease where there isn’t any.
There are several less dramatic implications of relying too much on AI in a health care context, such as ignoring the bigger picture of a patient’s health, weakening the trust in human physicians, and the management of ethics around use of data, to name a few.
Before we let our imaginations run wild, remember that the application of AI to real world problems on a grand scale is still in its early stages. There is still a long way to go, as evidenced by some funny stories that pop up, like the two Cornell chatbots conversing nonsensically [3], the Economist’s AI writing an article full of grammatically correct sentences that mean nothing [4], or IBM’s Watson being fed the urban dictionary by a well-meaning IBM engineer who simply wanted it to chat more colloquially [5].
At the same time, there are reports of AI outperforming human doctors on diagnoses [6,7] and predicting human lifespan with high accuracy [8]. These are serious claims, and demand significantly more scrutiny.
Not all tech revolutions are disruptive.
Not all tech revolutions are disruptive. They can be massively impactful and enhance the current state of affairs rather than replace it. The hope is that AI-based medical systems augment the ability of practicing GPs to make accurate clinical decisions and achieve better outcomes. For this reason, the scrutiny is warranted and necessary.
As biomedical engineers, we are trained to carefully consider the challenges inherent in any bank of patient data. How it was collected, how much noise exists and how the data is to be interpreted. And above all, in all cases, we must consider inter-patient variability and context. Inter-patient variability is unavoidable. Even though we are all similar, we are just different enough to make mathematical generalizations very difficult. Context – whether the data collection experiment was properly controlled, what the subject was doing when the data was collected and the premise under which it was collected – is critical. The absence of context or proper control of context allows bias to creep into the data itself.
The responsibility to avoid bias as much as possible must be on the development teams designing the models. I want to believe that companies like Google, Babylon and Ayasdi will do their best to ensure that responsible, robust design and testing are at the heart of their work. The FDA will have their say before anything makes it to the clinic, but work on human data is delicate, and it is difficult for a third-party auditor like the FDA to find the needles in all the haystacks (and with investing in AI going the way it is, there will quite literally be thousands of haystacks to go through). They look at clinical trial results and make the best judgement call they can. They have already permitted the marketing of a few AI-based medical devices this year – for detection of certain diabetes-related eye problems [9] and for use in neurovascular disease – and they are in the process of developing a guideline for this area of the field, via their Digital Health Innovation Action Plan [10].
That said, to quote a very logical, non-technical, non-medical expert:
“If it seems too good to be true, it probably is.” ~ My old man
Besides the inherent bias in the data, the developer’s bias is a significant threat to the training and testing of a machine learning model. Careful scientific method is mandatory, but it can be tempting to cut corners or lead the learning of our algorithm in the direction we want it to go. For example, if a model is trained to look at thousands of pictures of human faces and categorize them according to race, the lines are grey – who defines them and how? Add gender as a second dimension and the categorization becomes exponentially more difficult. In the case of physiological data, the challenge of categorization may be related to healthy and sick traits in cardiac activity (ECG), for example, or early markers of Parkinson’s disease in brain activity (EEG). Someone must define those categorizations very clearly, and that is difficult to do at times, even impossible in the worst-case. It is important to note that a large majority of data samples will be quite easy to define. It is in the edge cases that the success of a detection model is made or broken.
It is tempting to “feed the data into the model” and let it sort it all out. This is what the industry must avoid at all costs.
Dr Jean Gotman from the Montreal Neurological Institute is world-renowned for his EEG signal processing work in Epilepsy. His recommendation for a pattern detection algorithm to perform optimally in a true clinical setting, when we know very little about the incoming patient (which is exactly the criteria for AI in Health Care), is that its development should adhere strictly to the following rules [11]:
1. Training and testing data must be kept separate
The algorithm must be trained and tuned using one set of data, and it must be tested using another completely distinct set of data. If an algorithm parameter is adjusted based on the testing results or any behavior seen in the testing data, the testing data must be integrated into the training data and a new set of data must be tested. In the training of a machine learning model, variations of this recommendation can be considered as long as statistical integrity is maintained.
2. Data must be highly representative
Both the training and testing data sets must be as representative as possible of the final use case. This is the most difficult criteria to assess but it is of paramount importance. Otherwise the model will be tuned to a specific data set and will perform inadequately on generalized data. Ideally it should be collected from multiple sites using multiple systems.
3. Strict data inclusion / exclusion criteria must be followed
Data should be included as it becomes available and should only be excluded in cases that mimic real world clinical scenarios, such as corrupted or missing data, or sections of recordings that contain only incoherent information or during which data collection context was inappropriate for the use case. Criteria should never be adjusted to suit the data available. This is another significant mistake that would cause the model to perform poorly on generalized clinical data. Data should never be selected based on their features as they apply to the extraction and categorization.
4. Verification and validation must employ strict scientific method
During training and testing, data must be annotated in a scientifically and clinically responsible way by experienced professionals (rarely the same professionals as the developers). Detections true and false alike must be confirmed based on these annotations. During learning in the field, clinical results must be followed and plugged back into the model (e.g. in the life expectancy example, when finally used in a clinical context, how can accuracy be confirmed until the subjects actually die?).
5. Results should be assessed in context of the use case
If the model is designed to produce an alarm, for example, depending on the real-world use cases false alarms may be more of a problem than missed detections. Results will never be perfect, but the model must perform according to the intended application and not completely independently of context (i.e. highly accurately in any and all use cases, which is impossible). It helps if training and testing results are similar, as a strong result is also a robust one. If results do not reflect the real scenario given the data type and application, true positives and false negatives must be reviewed and classified to properly understand how well the clinical use case is reflected or will be affected. (Model robustness is a topic onto itself, one I would like to cover in another post.)
Model designers should also check their egos at the door. Poor results are as important as good ones. Being married to results will only lead to a biased model that will perform well on the bench and poorly on new clinical data as it arrives.
When done correctly, we all agree this has the power to change the world.
Let me be clear, I do believe there is enormous potential here to do good. AI has a natural fit in medical diagnostics. The ability to digest, process and interpret large amounts of past and present data in order to make a complex decision closely matches the task of the physician during a diagnostic intervention. But we must take the time and energy to do it responsibly and without ego to reap the true rewards.
As Rust and Marty contemplate light vs dark (arguably the main theme of the first season of the show), Marty determines that dark overpowers light, since the night sky full of stars is in fact more darkness than light. They conclude on a high note:
Rust: “You’re looking at it wrong, the sky thing.”
Marty: “How’s that?”
Rust: “Well, once there was only dark. You ask me, the light’s winning.”
Let’s hope Rust is right and that the AI movement does less harm than good. We have our work cut out for us and the stakes are high, but as in all great challenges the potential benefits are well worth it.
References:
1. Poole, David; Mackworth, Alan; Goebel, Randy (1998). Computational Intelligence: A Logical Approach. New York: Oxford University Press. ISBN 0-19-510270-3.
2. Laura Douglas, blog post : https://medium.com/@laurahelendouglas/ai-is-not-just-learning-our-biases-it-is-amplifying-them-4d0dee75931d.
3. News: Cornell two AI’s in a conversation: http://static6.businessinsider.com/this-is-what-happens-when-two-computers-talk-to-each-other-2011-8
4. News. Artificial intelligence. How soon will computers replace The Economist’s writers? https://www.economist.com/science-and-technology/2017/12/19/how-soon-will-computers-replace-the-economists-writers?fsrc=scn/li/te/bl/ed/howsoonwillcomputersreplacetheeconomistswritersartificialintelligence
5. News. IBM’s Watson swears like a sailor: https://www.businessinsider.com/ibm-watson-supercomputer-swear-words-2013-1
6. News. Bablyon AI: https://www.cnbc.com/2018/06/28/babylon-claims-its-ai-can-diagnose-patients-better-than-doctors.html
7. News. How one hospital used Ayasdi’s AI to improve clinical outcomes: https://medcitynews.com/2018/07/hospital-ayasdis-ai/
8. News. Google is Using Machine Learning to Predict the Likelihood of a Patient’s Death – with 95% Accuracy! https://www.analyticsvidhya.com/blog/2018/06/google-using-ai-predict-when-patient-will-die-with-95-accuracy/
9. News. FDA announces diabetes-related test: https://www.fda.gov/newsevents/newsroom/pressannouncements/ucm604357.htm
10. FDA – Digital Health Innovation Action Plan: https://www.fda.gov/newsevents/speeches/ucm605697.htm
11. Gotman J, Flanagan D, Zhang J, Rosenblatt B. Evaluation of an automatic seizure detection method for the newborn EEG. Electroencephalography and Clinical Neurophysiology. 1997; 103: 363-369